Connettiamo i dati con le persone, i prodotti e i progetti
Azienda?
Trova un talento
Candidato?
Trova opportunità di carriera
Più di 35 aziende in tutto il mondo
specializzazione in ambito STEM
di recruitment
About us
Chi siamo
Con sede a Londra, OSN è un’innovativa società specializzata nel recruiting di profili STEM(A) italiani di livello globale, che si identifica come una boutique di talenti. Dal 2013 uniamo infatti l’imprenditorialità della City con la passione e la tenacia di un team esperto di headhunter interamente italiano.
Ci posizioniamo così come partner affidabile per molte aziende italiane alla ricerca di talenti internazionali, anche grazie alla nostra trasformazione in full liquid company da marzo 2020.
Ad oggi abbiamo lavorato con eccellenze di diversi ambiti e settori dell’economia italiana e nel 2017 abbiamo anche collaborato con il team di Diego Piacentini, allora Commissario per l’Agenda Digitale del Governo Italiano.
Grazie a questo doppio volto, riusciamo a intercettare gli italian brains all’estero interessati a valutare un ritorno in Italia.
Da oltre 10 anni, abbiamo compreso che il modello tradizionale di recruitment non funziona più per i profili innovativi.
SCENARIO
Cosa dicono i numeri
Le indagini del Sistema informativo Excelsior sulle previsioni dei fabbisogni occupazionali in Italia (2023-2027) di Unioncamere hanno evidenziato come le imprese facciano molta fatica a trovare candidati con competenze digitali e nelle aree STEM.
Infatti, i principali macro-trend stanno già ridefinendo il mercato del lavoro e avranno un impatto sempre più marcato nei prossimi anni.
Le transizioni tecnologiche e ambientali aumenteranno la richiesta di competenze STEM, green e digitali, amplificando lo “skill gap” nel mercato del lavoro.
Tra il 2023 e il 2027, saranno necessarie competenze green per quasi 3,9 milioni di lavoratori e più di 2 milioni di occupati dovranno possedere competenze digitali (oltre il 56% del fabbisogno totale). In particolare, nelle aree STEM, le carenze più critiche emergono nelle scienze matematiche, fisiche, informatiche e ingegneristiche.
Azienda?
Trova un talento
Candidato?
Trova opportunità di carriera
cosa facciamo
Headhunting
In OSN reclutiamo e posizioniamo team di talento e profili senior/executive per aziende, startup tecnologiche ed enti governativi. Abbiamo creato una community internazionale di oltre 30.000 membri composta da Data Scientist, Data Engineer, Devops, Researcher, Cyber Security Expert, Consultant e Director. Siamo l’unica società di recruitment che fa leva su metodi di reclutamento progettati su misura per il settore dei dati.
testimonianze
Chi crede in noi?
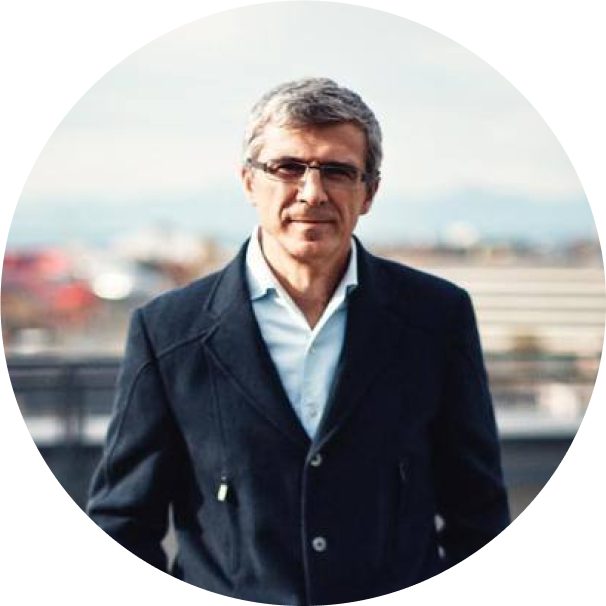
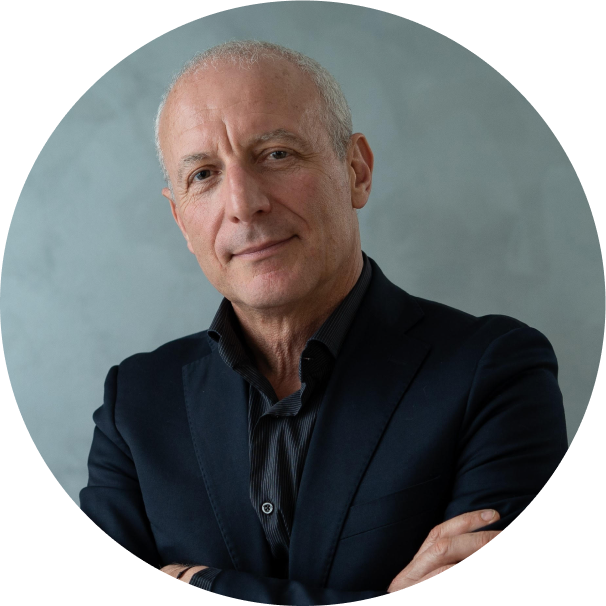
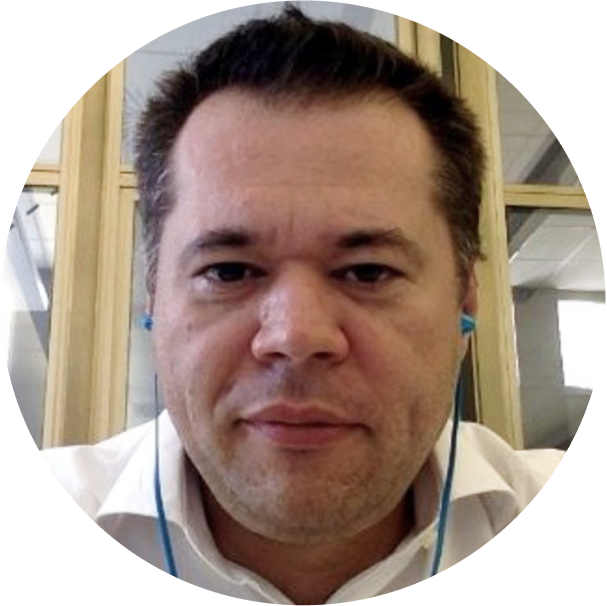
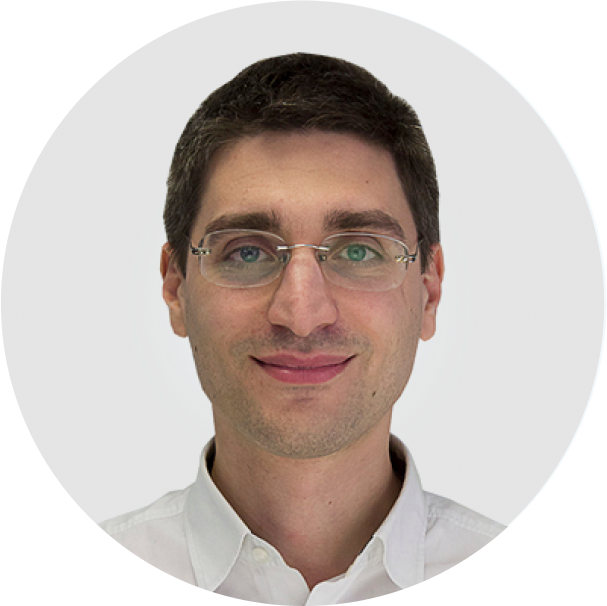
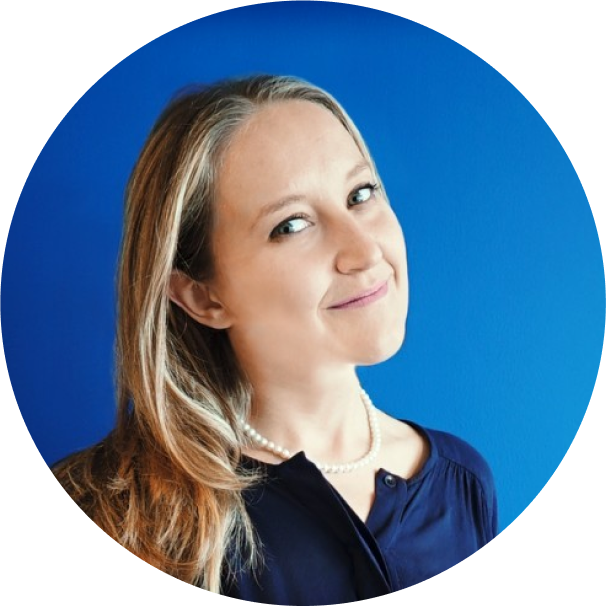
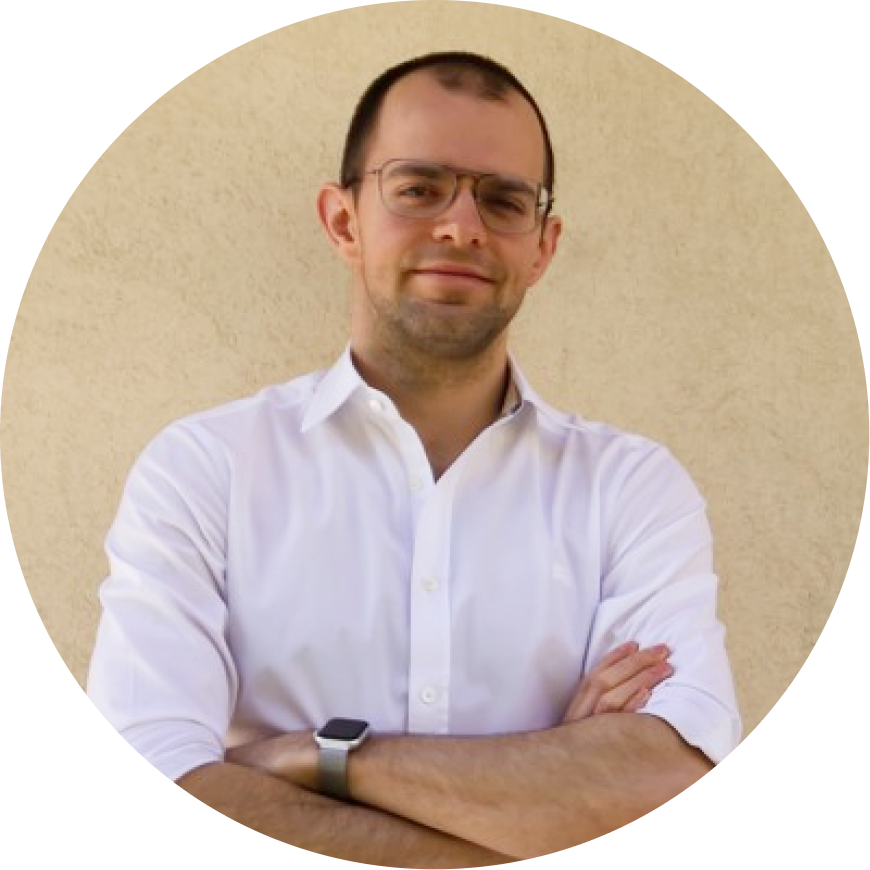
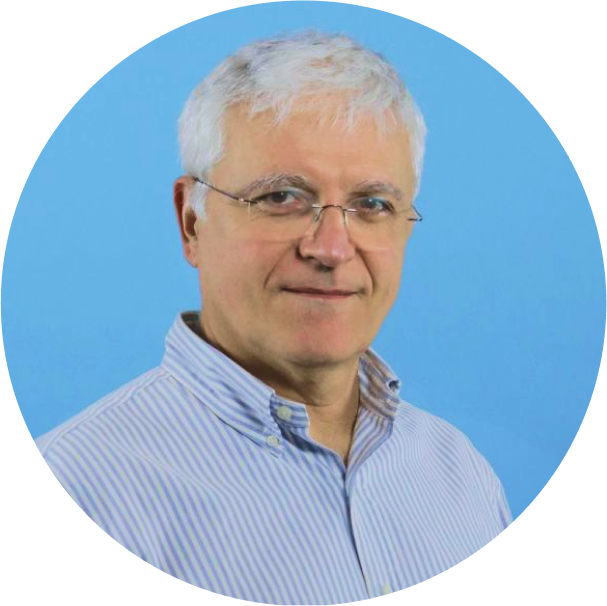